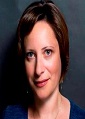
Yulia Einav
Holon Institute of Technology, Israel
Title: Thalassemia minor diagnostics by a computational method
Biography
Biography: Yulia Einav
Abstract
Thalassemia minor (TM) condition is often unrecognized and undiagnosed due to technical or cost issues. The benefit of early diagnosis of TM patients is a prevention of unnecessary treatments and costly tests and a referral to a genetic counseling. The use of complete blood count (CBC) parameters to screen for TM condition has been proposed in the past decade and performed with variable success. Here, we propose to use artificial neural networks (ANNs), which are successfully applied to various fields of medicine and beyond to find patterns in ambiguous data. ANN model comprises of an interconnected group of artificial neurons that learn through experience. Like a biological neuron network, the ANN is designed to obtain an input, analyze the data and supply the output, which in most cases would be some prediction about the patterns and behavior of the studied system. In this study, we created more than 1,500 ANNs and selected the networks that gave highest accuracy results. Input consisted of six CBC parameters: MCV, RBC, RDW, HB, MCH, and platelet count. This broad approach allowed us to provide a differential diagnosis for TM patients with specificity above 0.96 and sensitivity of 1. In addition, we show that in our method MCV, RBC, and RDW counts are of greater importance than the other three parameters. The low cost of our model makes it important worldwide and especially in the developing countries. Moreover, similar ANN-based method can be applied for the screening of other diseases that change CBC parameters.
Speaker Presentations
Speaker PPTs Click Here